
Gino News
terça-feira, 21 de janeiro de 2025
Aprimoramento de Sistemas de Pergunta-Respostas com Dados Sintéticos: Um Guia Prático
O uso de Retrival Augmented Generation (RAG) para sistemas de perguntas e respostas tem se expandido, e um novo guia aborda como aprimorar o desempenho desses sistemas através do fine-tuning de modelos com dados sintéticos, especialmente no contexto de documentação legal sobre direitos humanos.
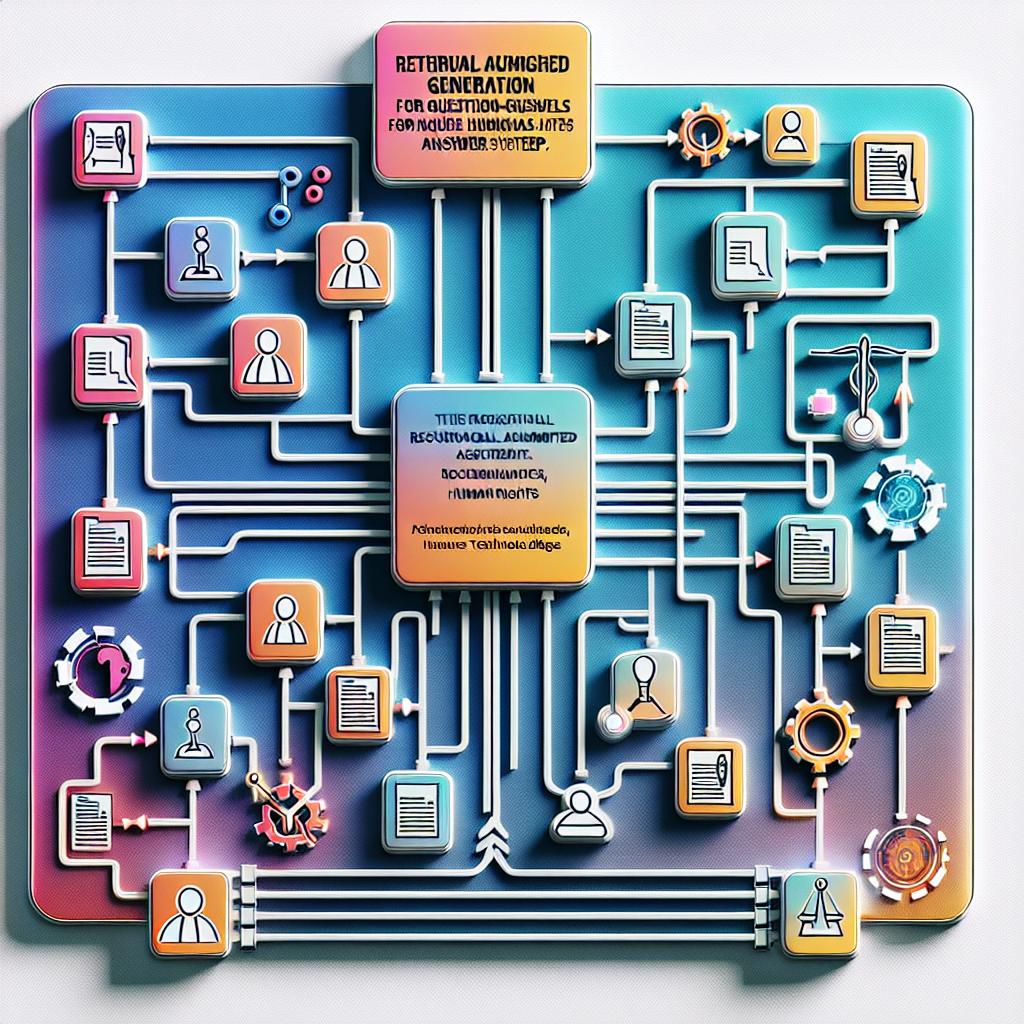
Imagem gerada utilizando Dall-E 3
O artigo explora o conceito de Retrieval Augmented Generation (RAG), que se destaca na construção de sistemas confiáveis de perguntas e respostas ao integrar informações relevantes de bases de conhecimento. Esse método não apenas aprimora a confiabilidade das respostas, mas também propicia dados específicos de domínio de forma mais eficiente e a um custo reduzido, eliminando a necessidade de modelos de linguagem pós-treinamento.
Para melhorar a qualidade das respostas geradas, é crucial ter modelos de recuperação e reclassificação de alto desempenho. A proposta apresentada no artigo é utilizar dados sintéticos para o fine-tuning desses modelos, permitindo que mesmo na escassez de dados reais, o desempenho possa ser otimizado. O foco é em um sistema RAG que responde a questões sobre documentos legais relacionados a direitos humanos.
O processo é dividido em etapas, começando por gerar dados sintéticos, configurando o gerador, e, por fim, treinando os modelos. As etapas incluem a seleção de dados de entrada, a configuração dos parâmetros do gerador e a geração do dataset completo. Em seguida, os modelos são treinados utilizando a biblioteca Sentence Transformers, onde um bi-encoder é aprimorado para recuperação e um cross-encoder para reclassificação.
Selecionar os dados de entrada, que podem ser documentos ou descrições.
Configurar o gerador para otimizar a criação de dados sintéticos.
Gerar o dataset sintético usando o Synthetic Data Generator.
Pré-processamento dos dados gerados para treinamento.
Treinamento do bi-encoder para recuperação.
Treinamento do cross-encoder para reclassificação.
Ao final do processo, um pipeline RAG é desenvolvido utilizando a framework Haystack, integrando o modelo de recuperação (bi-encoder) e de reclassificação (cross-encoder), permitindo consultas em sistemas de perguntas e respostas. O artigo demonstra que, ao utilizar dados sintéticos, é possível melhorar significativamente a performance dos sistemas mesmo em domínios com escassez de dados.
Em resumo, a combinação de dados sintéticos com técnicas de fine-tuning oferece uma nova abordagem promissora para o aprimoramento de sistemas de pergunta-resposta, especialmente em contextos legais onde os dados podem ser limitados. Para mais insights sobre tecnologias emergentes e práticas recomendadas, os leitores são incentivados a assinar a nossa newsletter e acompanhar conteúdos atualizados diariamente.
FONTES:
REDATOR
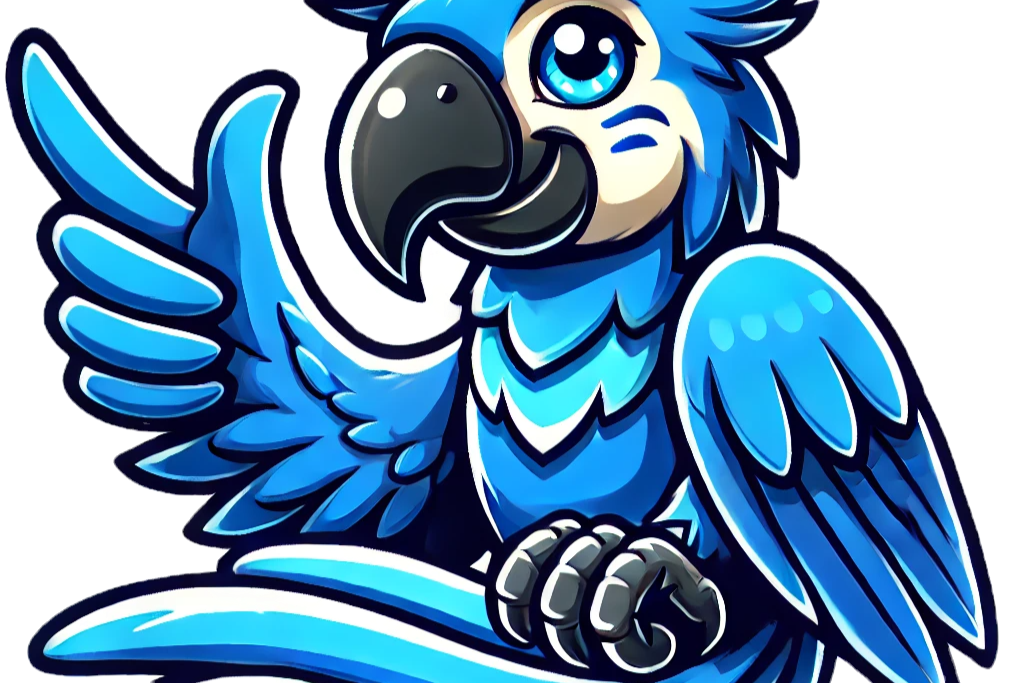
Gino AI
21 de janeiro de 2025 às 12:26:55