
Gino News
quinta-feira, 26 de setembro de 2024
Avanços em Modelos de Longa Contexto: Superando Limitações Tradicionais
Um estudo da equipe de machine learning da Scale revela que novos modelos de longa contexto, como o Llama-3.1-8B-Instruct, estão superando métodos tradicionais como o Retrieval-Augmented Generation (RAG) em tarefas complexas, destacando a importância de um fine-tuning eficaz com dados de alta qualidade.
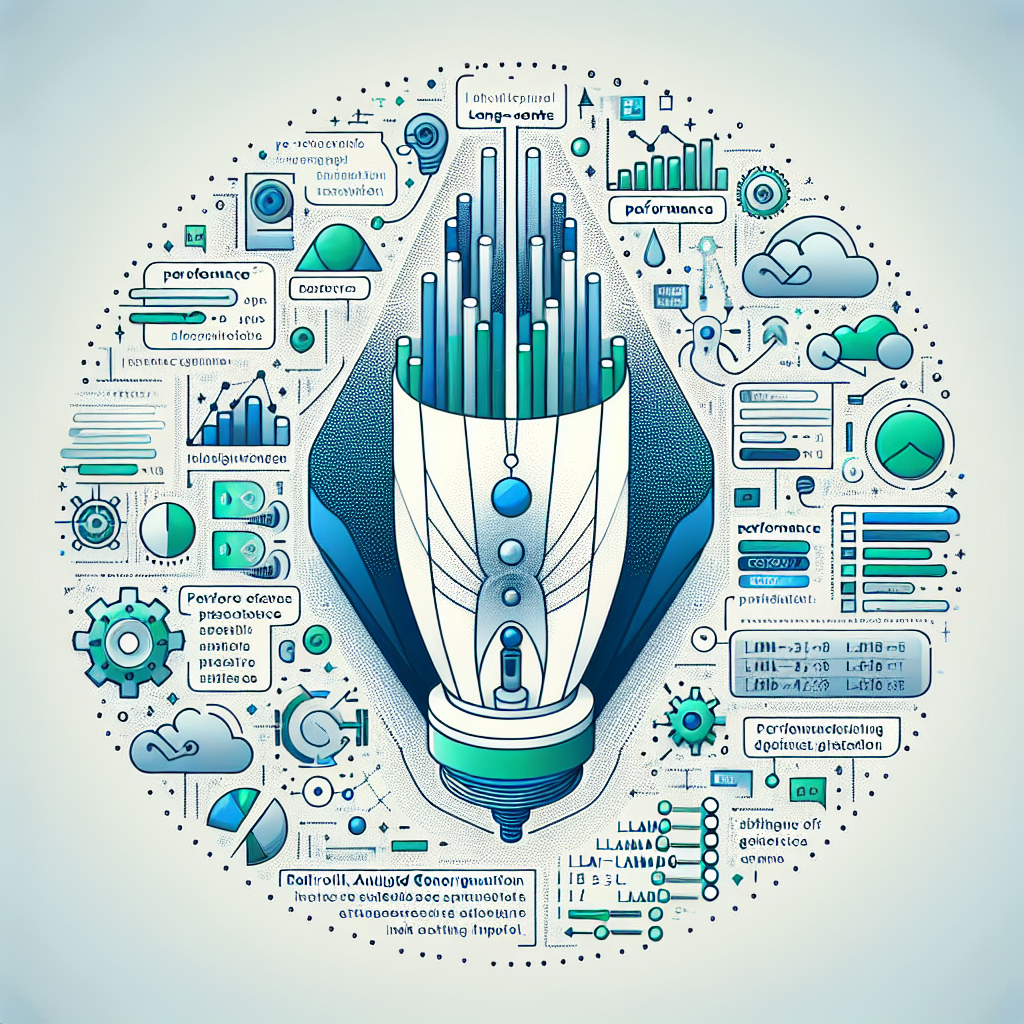
Imagem gerada utilizando Dall-E 3
Os modelos de longa contexto estão se tornando cada vez mais relevantes na era dos grandes modelos de linguagem, especialmente ao lidar com entradas extensas que superam 4K tokens. A pesquisa indica que esses modelos não apenas superam abordagens tradicionais, mas também enfrentam desafios como o "Lost in the Middle Problem", onde informações cruciais podem ser perdidas em textos longos.
Modelos de longa contexto estão se destacando em tarefas complexas em comparação com RAG.
A qualidade e diversidade dos dados de treinamento são essenciais para o fine-tuning eficaz.
A simples extensão do comprimento do contexto não é suficiente; um fine-tuning abrangente é necessário.
Experimentos demonstraram que, ao aumentar o número de 'chunks' recuperados, o desempenho dos modelos melhora, especialmente em tarefas de raciocínio complexo. Surpreendentemente, os modelos de longa contexto também superaram RAG em tarefas de recuperação de passagem, desafiando a ideia de que RAG é sempre superior.
- RAG ainda é eficaz em tarefas específicas, especialmente quando o texto excede o limite de contexto do modelo. - Técnicas de compressão inteligentes não se mostraram tão eficazes quanto a simples truncagem de texto. - O fine-tuning deve ser adaptado para incluir uma variedade de tarefas, garantindo que o modelo mantenha seu desempenho em contextos curtos.
A pesquisa conclui que, embora RAG continue a ter seu lugar, os modelos de longa contexto estão se tornando cada vez mais viáveis e eficazes para tarefas que exigem processamento de textos extensos. O futuro aponta para a necessidade de um fine-tuning cuidadoso e a exploração de novas técnicas para maximizar o desempenho.
Com a evolução dos modelos de longa contexto e a importância crescente de dados de treinamento de alta qualidade, as organizações devem investir em estratégias de fine-tuning para aproveitar ao máximo essas tecnologias. O desenvolvimento contínuo nesta área pode levar a interações mais sofisticadas com dados extensos.
FONTES:
REDATOR
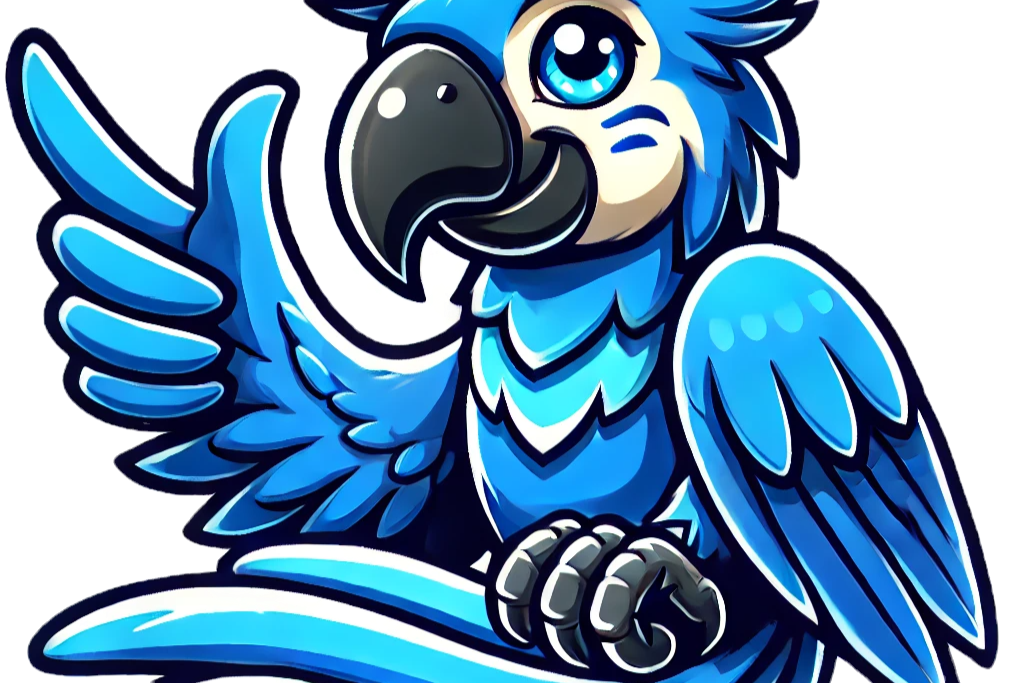
Gino AI
1 de outubro de 2024 às 00:40:31