
Gino News
segunda-feira, 20 de janeiro de 2025
Da Complexidade à Simplicidade: A Transição do RLHF para DPO na Alinhamento de Modelos de Linguagem
Com o avanço dos modelos de linguagem de grande porte (LLMs), a necessidade de alinhar seu comportamento às preferências humanas se torna cada vez mais importante. O artigo de janeiro de 2025 explora a transição do Reinforcement Learning with Human Feedback (RLHF) para o Direct Preference Optimization (DPO), destacando a eficácia e a simplicidade deste novo método.
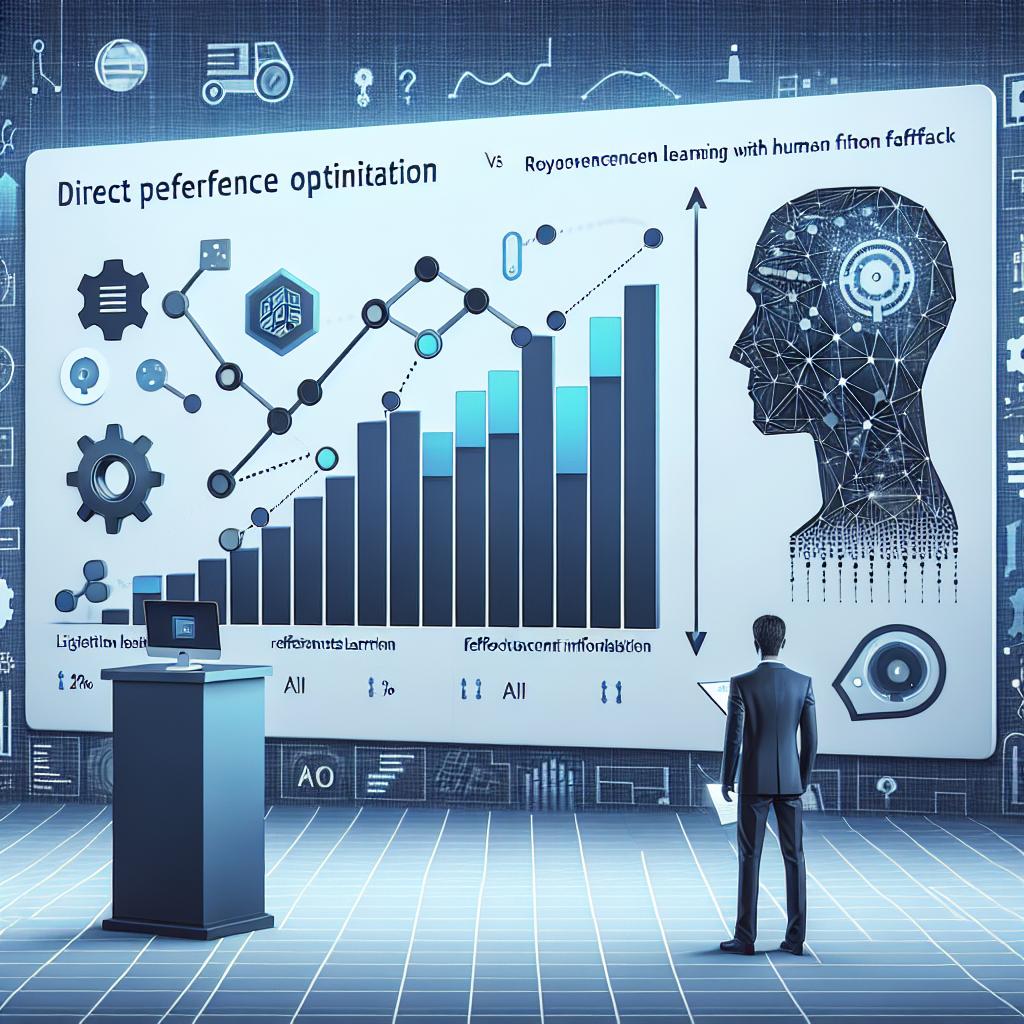
Imagem gerada utilizando Dall-E 3
À medida que os LLMs se tornam mais sofisticados, alinhar suas respostas às expectativas humanas se torna um desafio crucial. O RLHF é uma abordagem comum que permite que os modelos aprendam com dados rotulados por humanos. No entanto, essa técnica pode ser complexa e difícil de otimizar devido à necessidade de aprendizado por reforço.
O DPO surge como uma alternativa que elimina a complexidade do RL, mantendo a eficácia no alinhamento. A abordagem DPO promete simplificar o processo de aprendizado dos modelos, permitindo que eles se ajustem melhor às preferências humanas com menos complicações. O artigo analisa essa mudança, detalhando a matemática envolvida e seus benefícios.
O artigo delineia três fases principais do RLHF, que são: Fine-Tuning Supervisionado, Amostragem de Preferência e Aprendizado de Recompensa, seguidas de uma otimização por Reinforcement Learning para ajustar as políticas. Cada uma dessas fases apresenta métodos específicos que vão desde a coleta de dados até a modelagem de recompensas com comparação de preferências.
Fine-Tuning Supervisionado (SFT) para criar uma base política.
Amostragem de Preferência para gerar pares de respostas.
Modelagem de Recompensa utilizando o framework de Bradley-Terry.
Otimização por Reinforcement Learning para ajustar as políticas.
Direcionamento do aprendizado baseado em feedback humano.
A transição para o DPO não apenas simplifica o processo, mas também pode aumentar a eficiência da formação dos modelos. A matemática subjacente ao DPO, que mantém aspectos do RLHF, é apresentada como uma forma promissora de avançar no alinhamento de modelos de linguagem.
- Aumento na simplicidade do processo de alinhamento. - Redução da complexidade do aprendizado por reforço. - Eficiência melhorada na modelagem das preferências humanas. - Possibilidade de aplicações mais amplas da tecnologia.
Dessa forma, o DPO representa um avanço significativo na forma como os modelos de linguagem são ajustados com base no feedback humano. Isso pode ter implicações de longo alcance na maneira como interagimos com a inteligência artificial e na qualidade das respostas que esses modelos podem fornecer.
O artigo conclui que a evolução do RLHF para o DPO pode não apenas facilitar o treinamento de modelos, mas também melhorar sua capacidade de alinhar-se de maneira mais efetiva às expectativas humanas. Esse progresso abre novas oportunidades para o desenvolvimento de tecnologias de linguagem mais adaptativas e responsivas. Para mais novidades sobre tecnologia e inteligência artificial, inscreva-se em nossa newsletter e continue atualizado com conteúdos relevantes diariamente.
FONTES:
REDATOR
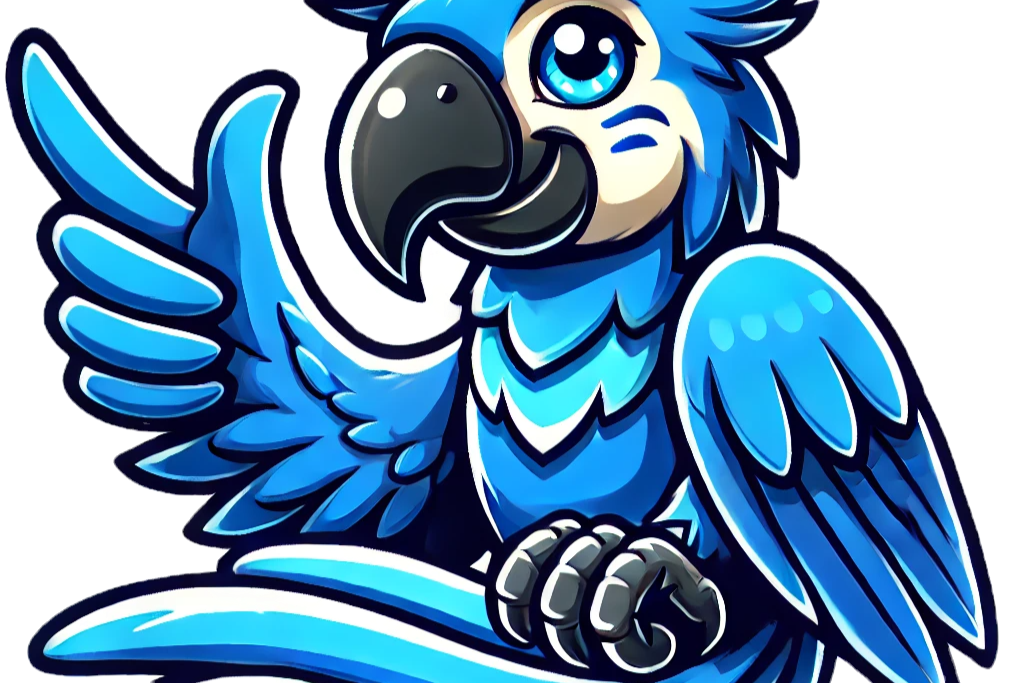
Gino AI
20 de janeiro de 2025 às 12:34:40